People often think about transcription and voice commands when they think of voice AI. Those engines focus on the content. However, Speaker Recognition
recognizes the speaker rather than the speech. Yet, language is a critical determinant of the performance of Speaker Recognition
. The phonetic variability across languages affects the success rate of Language-Dependent Speaker Recognition
software. The performance degrades when there is a language mismatch between training and testing data.
What’s Language-Dependent Speaker Recognition?
Language-Dependent Speaker Recognition
is effective when users speak a particular language or dialect because researchers train algorithms with those language in mind. Thus, Language-Dependent Speaker Recognition
may fail to recognize users speaking a different language. For example, Speaker Recognition
software, trained for Australian English, may have lower accuracy in another language or dialect, such as German or Scottish.
The variety of human voices and unique sounds of each language makes removing Language Dependency
challenging. For example, Microsoft still supports only eight languages for its Speaker Recognition engine. However, Language Dependency
does not fit into today’s global world. More than half of the population speaks at least two languages. Enterprises operate in multiple countries and serve users across the globe.
What’s Language-Independent Speaker Recognition?
Researchers train Language-Independent Speaker Recognition
models using diverse speech corpora across languages and dialects, removing language dependency. Thus, Language-Independent Speaker Recognition
models serve any speaker, not just those who speak selected languages and dialects. However, when the training data is not diverse, the performance of speaker recognition software in specific languages varies across languages. The closer the DET curves, the more robust speaker recognition engines are across languages.
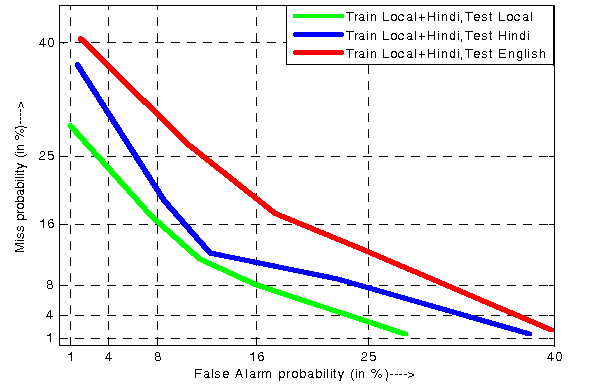
Figure 1: DET Curve of a speaker recognition engine trained, performance degrades when there’s a language mismatch between training and testing data.
A multilingual speech database for speaker recognition
The chart above depicts the performance of a Speaker Recognition
engine trained on a dataset that does not include English. It degrades significantly due to the language mismatch when tested with the English test data, compared to the other two languages from the training dataset.
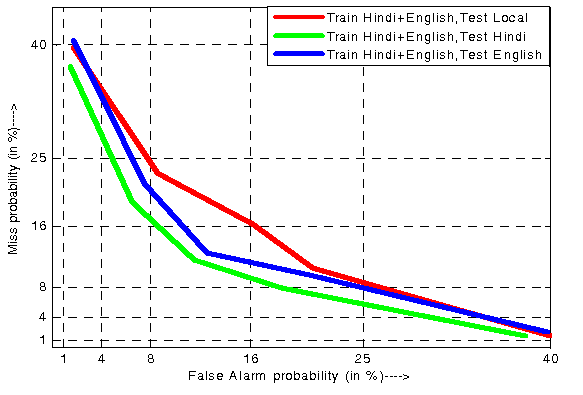
Figure 2: DET Curve of a speaker recognition engine trained, performance degrades relatively less when there’s a language mismatch between training and testing data
A multilingual speech database for speaker recognition
The chart above depicts the performance of a Speaker Recognition
engine trained using a dataset that does not include the Local language. It doesn’t degrade as much as in the previous chart. The curves are closer to each other, meaning this engine is more robust than the first one. We expect curves to overlap if the engine is 100% Language-Independent
.
Please note that Language-Dependency
does not refer to the measure of performance. While it suits better to many applications, Language-Dependent Speaker Recognition
can perform better than Language-Independent Speaker Recognition
and vice versa.
Picovoice’s Speaker Recognition, Eagle, is Language Independent
. Start building for free and serving users across the globe.