One can find hundreds of websites comparing different Speech to Text
software when they google Speech to Text
alternatives. As if the choice overload is not already a big problem, most comparisons mix speech technologies. You can see Google Cloud Speech-to-Text compared against Dragon Speech Recognition Software, Microsoft Bing Speech API, and even Krisp. It’s like comparing apples to oranges.
Speech to Text
is the most well known Speech Recognition software. That’s why it’s unsurprising that most people think of Speech to Text
upon hearing Speech Recognition
. It’s a go-to choice not just for those new to the field and even for vendors and researchers. Hence, various applications, from dictation to keyword search and domain-specific voice assistants, integrate Speech to Text
. Similarly, enterprises think they should get an open-source Speech to Text
engine and customize it accordingly for their needs. It’s one of the most common reasons why voice AI projects fail. Although results look similar, the right Speech Recognition
software allows developers to build more accurate, responsive, and efficient products with higher performance. Speech to Text
has three well-known limitations that affect the performance of voice products:
- Out-of-vocabulary
- Homophones
- Decoding time
When any of them affects the product performance, one should look for Speech to Text Alternatives (STTA)
.
What’s the Out-of-Vocabulary Problem in Speech to Text?
Out-of-Vocabulary (OOV)
refers to words with no or low occurrence in the training data. Like machines, we also struggle with understanding and writing the words we hear for the first time. Remember when your friend shared their address with a street name that you never heard of before or met a new person with a unique name? They are usually operating words, so we ask people to repeat or spell them, so we can learn on the spot. Speech to Text
doesn’t have a chance to ask to repeat or spell the word that is not in its vocabulary.
Proper nouns are a well-known out-of-vocabulary problem for Speech to Text
. If your use case requires capturing proper nouns, you should look for custom Speech to Text
for transcription and Speech-to-Index for keyword search.
Read more if you’re interested in a Speech to Text Alternative for Search!
What’s the Homophones problem in Speech to Text?
The problem of homophones in Speech to Text
refers to the difficulty in accurately transcribing words that sound the same but have different meanings and spellings. Homophones are words that are pronounced similarly but have different definitions, such as "two," "to," and "too."
Humans struggle with homophones too. If you watch the Simpsons, you’ll remember the Spellympics from S14E12 “I’m spelling as fast as I can.” The moderator shares the word weather, and the contestant asks for an example sentence. The moderator responds: “ I don't know whether the weather will improve.”
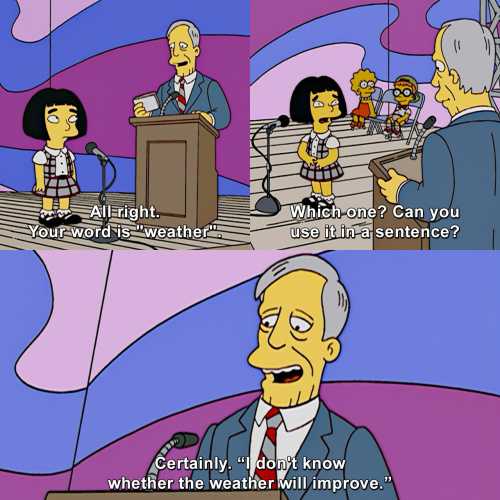
Cartoon from tvgag.com
In most cases, humans naturally differentiate homophones given the context. While listening to a recipe, we do not mix “flour” with “flower.” However, generic Speech to Text
engines are unaware of specific domains and contexts. If you’re working on a domain-specific application, you should look for custom Speech to Text
for transcription and Speech-to-Intent for voice commands.
Read more if you’re interested in improving the accuracy of voice-controlled solutions with Speech to Text Alternative for Voice Assistants!
What’s the decoding time in Speech to Text?
Decoding time refers to the time Speech to Text
spends processing and transcribing spoken words into written text. It encompasses the various computational steps to convert audio input into text output. The decoding time depends on several factors, such as the complexity of the acoustic and language models, the computational resources available, and the length of the input audio. Decoding time doesn’t affect the performance of a product while transcribing a file, such as an interview or podcast. However, it matters for time-sensitive applications enabling voice activation and voice control. Decoding time is one of the reasons why Speech to Text is not for wake word detection. If the responsiveness of an application is crucial, experiment with Keyword Spotting and Speech-to-Intent engines. If you still need Speech to Text
, opt for on-device streaming Speech to Text
engines like Cheetah. Cheetah Streaming Speech-to-Text is the most efficient real-time Speech to Text
solution available. It removes network latency and minimizes decoding time.
Knowing the differences among various speech technologies and when to use Speech to Text
or Speech to Text Alternatives
significantly affect the success of products in most cases. Contact Picovoice’s Consulting Services if you need help choosing Speech to Text
or a Speech to Text Alternative
.