Speaker Diarization
, or simply Diarization
, is figuring out “who spoke when?”. In practice, it’s about “who spoke when and what?”. Let me expand. In academia, Speaker Diarization is the problem of partitioning a stream of audio into segments spoken by single speakers. But Speaker Diarization is usually a subcomponent of speech-to-text systems. It increases readability. Speaker Recognition
, Speaker Identification
, and Speaker Clustering
(can) refer to the same technology.
If you need Speaker Diarization, you have to make a strategic decision between build, open-source, and buy. This decision has long-term implications for the product, resource allocation, and budgeting.
Build
There are two dominant paradigms for building a diarization system. One is based on speaker embeddings generated by a (possibly deep) model and then applying clustering on top to create partitions. Speaker Diarization with LSTM is an example of this method. Alternatively, one can redefine diarization as a classification problem and solve it end-to-end. End-To-End Speaker Diarization Conditioned on Speech Activity and Overlap Detection is an example of an end-to-end approach. Although the latter has the potential to outperform the clustering-based, it is much harder to train and requires significantly more labelled data.
Open-Source
pyannote.audio is an open-source Speaker Diarization project. Also, speech-to-text projects such as Kaldi or SpeechBrain have a diarization subcomponent embedded in their framework.
Buy
There was no standalone Speaker Diarization commercial API, until Picovoice introduced Falcon Speaker Diarization. Developers needed to use one of the speech-to-text (STT) APIs and enable Speaker Diarization. This option can cost extra on top of STT API cost. The downside of API-based offerings is that they are unbearably expensive as your business scales.
Azure Speech-to-Text, AWS Transcribe, Google Speech-to-Text, and IBM Watson Speech-to-Text offer Speaker Diarization. The cost is between $1 to $3 per hour. Besides cost, STT vendors treat Speaker Diarization as a feature that exists or not without communicating its performance. Picovoice’s open-source Speaker Diarization benchmark shows the performance of Speaker Diarization capabilities of Big Tech STT engines varies.
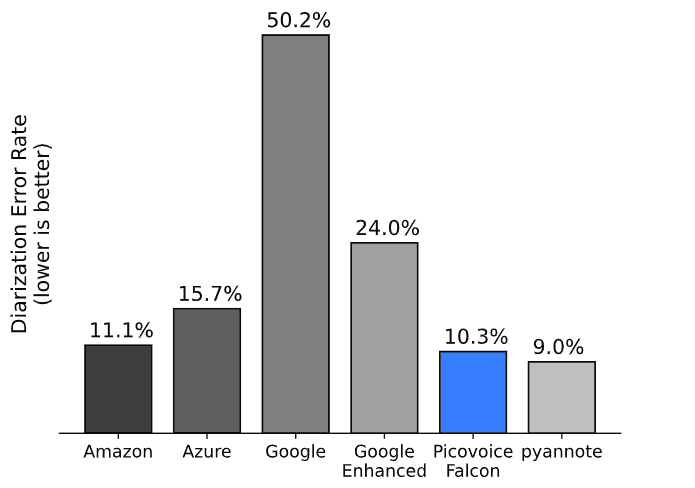
Also, there is a flow of SaaS startups in speech recognition. Speechmatics, AssemblyAI, Deepgram, and Rev are to name a few. They might have better technology, or you might be able to negotiate a better deal.