Spoken Language Understanding
(SLU) sits at the intersection of speech recognition and natural language processing. Spoken Language Understanding
focuses on extracting meaning from speech.
The conventional SLU
approach processes spoken utterances by applying two distinct components sequentially. First, Speech-to-Text (STT)
transcribes speech data, and then Natural Language Understanding
(NLU) extracts meaning by processing the transcribed text. Voice assistants Alexa and Google Assistant use this approach. The accuracy of conventional SLU
relies on the performances of independently trained STT
and NLU
modules. Erroneous STT
outputs lead to incorrect NLU
predictions.
The modern SLU
approach uses an end-to-end and jointly-optimized model instead of two distinct components. Fusing STT
and NLU
removes the cascaded errors. Picovoice’s Rhino Speech-to-Intent
uses the modern SLU
approach. Picovoice coined the term Speech-to-Intent
as Rhino directly infers intents from speech without converting it to text. Amazon calls this technology FANS, which stands for Fusing ASR and NLU for SLU.
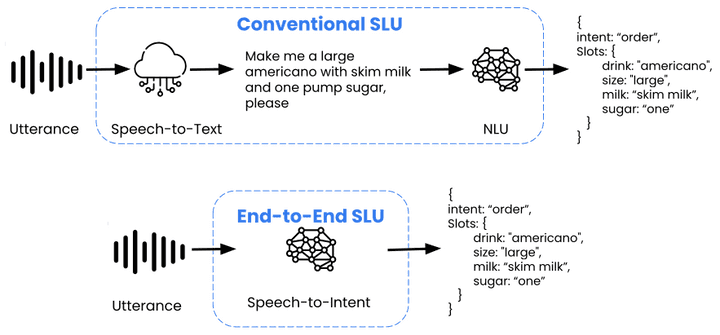
How does SLU differ from NLU?
In summary, both SLU
and NLU
are focused on understanding natural expressions in natural language. While SLU
focuses on speech, NLU
focuses on text input. SLU
uses NLU
whether it’s trained independently or jointly with speech recognition.
SLU
has gained more popularity with the recent advances in deep learning. The query “spoken language” returns over 1000 studies on both Amazon and Microsoft research publications websites. Given the performance and accuracy benefits of the modern approach, it is not surprising. Below is the accuracy comparison chart of Rhino (end-to-end) and alternatives (conventional).
Conventional or modern SLU?
The answer to this question depends on the availability of corpora and information. If there’s enough of it, then the answer is modern end-to-end SLU
. It offers improved performance and accuracy over traditional cascading SLU
. However, finding corpora in the target language and domain is not easy. Therefore, for open-domain use cases such as voice assistants like Alexa, traditional cascading SLU
works better considering the variety of topics. This is mainly because text-based NLU
has been around longer than speech-based NLU
and has richer datasets.
However, for domain-specific, context-aware enterprise voice assistants such as IVR systems, the modern end-to-end SLU
is preferable. One would have enough data and domain expertise to train them. Eliminating STT
errors and training the models specific to the domain improves the performance of voice products. While interacting with a telehealth app, one wouldn't say “upright his” but “arthritis.” Read Picovoice’s strategy guide to select the best engine for your use case or contact the Picovoice Consulting team and work with experts from strategy to execution.