Every voice AI vendor, including Picovoice, has an article on what WER
means and how to calculate it. It’s an important metric to compare the accuracy of speech-to-text (STT) engines. However, WER
alone does not mean much. WER
is easy to manipulate. Vendors can share technically correct but misleading claims. It’s critical for an enterprise to know the nuances and calculate a WER
by using its own data.
1. WER varies across different datasets.
If a vendor provides you with a single number when you ask for their WER
without any information on the dataset, it’s not a good sign. There is no single WER
figure for any STT. WER
depends on the dataset.
The same STT can return different word error rates when the text is read by other speakers or by the same speaker in different environments. The recording quality, microphone quality, background noise, proper nouns and industry-specific terms affect the WER
.
For example, Picovoice can claim WER
of its Speech-to-Text, Leopard is 5.39% - which is 100% accurate, yet misleading. Leopard’s WER
could be 9%, 12% or 17% based on the dataset.
Picovoice publishes open-source benchmarks frameworks that help enterprises run benchmarks instead of just providing a word error rate.
2. When a speech-to-text engine outperforms another, it doesn’t necessarily mean it could continuously outperform that alternative.
Let’s add Google Speech-to-Text and IBM Watson Speech-to-Text to the chart above.
IBM Watson Speech-to-Text can claim that it outperforms Google Speech-to-Text, considering the results of LibriSpeech test-clean and TED-LIUM. Google Speech-to-Text can claim the same, considering the results of LibriSpeech test-other and CommonVoice.
An engine that works well for one use case doesn’t necessarily work for others with the same accuracy. Enterprises should run benchmarks with their dataset.
3. Vendors can manipulate WER even by using the same dataset.
The first two points illustrated why one should use its dataset to calculate WER
. Most voice AI vendors will tell you that. Some will ask for data to provide you with a benchmark, including various vendors - which is great, right? Instead of you doing the work, someone else will do it. Be cautious! They may fine-tune their model to improve the accuracy of their ASR while using the generic model for others.
4. In real life, every error doesn’t have the same weight.
WER
is a generic method and treats all errors equally. It doesn't assign a higher weight when the error changes the meaning of the sentence or priority words. It’s critical to check the errors to determine how important they are for your use case. Speech-to-text with a lower WER
may systematically miss some essential words for your use case. If a speech-to-text engine with a lower WER
keeps missing the names of your products, then you’ll probably not be happy with its accuracy.
5. Fine-tuning models can improve the Word Error Rate.
It’s a fact that there is no 100% accurate speech-to-text engine. However, models can be fine-tuned for specific use cases, resulting in a lower WER
, i.e. higher accuracy. Some voice AI vendors use customer data with or without customers’ explicit consent to improve their models.
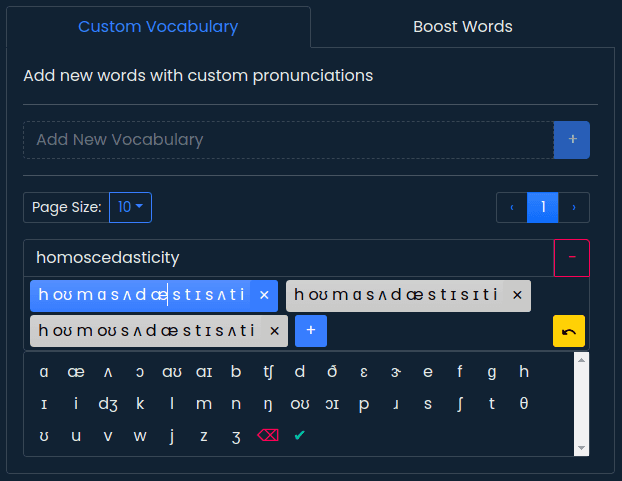
On the other hand, Picovoice does not have access to customer data. It provides customers with a self-service console to fine-tune the models without sacrificing privacy. Above is a screenshot of Picovoice Console, where users can add words specific to their use case by using their IPA translation to train speech-to-text models. Improve speech-to-text accuracy, or start building now!
Start Building